Twenty-Third Annual Post-Graduate Summer School
ACT-R 2016 Post-Graduate Summer School
John R. Anderson and Christian Lebiere
Psychology Department, Carnegie Mellon University
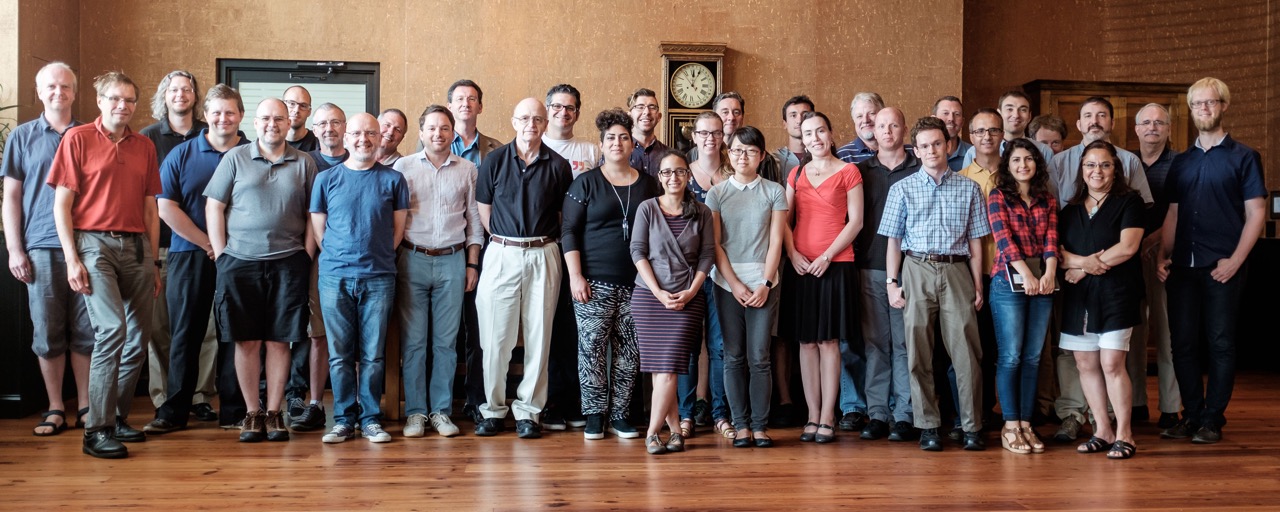
Attendees of the 2016 ACT-R Post-Graduate Summer School from left to right (and front to back when one directly behind the other): Marc Halbrügge, Niels Taatgen, Robert Thomson, Christopher Stevens, Dan Bothell, Jelmer Borst, Wayne Gray, David Peebles, Michael Martin, David Reitter, Christian Lebiere, John Anderson, Andrea Stocco, Burcu Arslan, Michael Collins, Othalia Larue, Trudy Buwalda, Robert West, Qiong Zhang, Cvetomir Dimov, Bella Veksler, Troy Kelley, Glenn Gunzelmann, Matthew Kelly, Kevin Gluck, Ion Juvina, Farnaz Tehranchi, Vladislav Veksler, Frank Ritter, Cleotilde Gonzalez, Lael Schooler, Bill Kennedy, Harmen de Weerd.
The 2016 ACT-R Post Graduate Summer School took place from August 7 to 9, 2016 at the Cork Factory Hotel in Lancaster, Pennsylvania. Abstracts from the talks are provided below, and the slides from many are linked from the title.
Jelmer Borst (Groningen) – ACT-R and Neuroscience Revisited: What Did We Learn from EEG and MEG?
Since the early 2000s, we have been using fMRI as a means of informing ACT-R models. As a direct result, the imaginal buffer was introduced to the architecture. After the mapping between ACT-R modules and brain regions became more established, fMRI could also be used as a means of testing and constraining ACT-R models, in some cases requiring a significant redevelopment of models. The drawback of fMRI is its low temporal resolution. To approach the level of temporal detail of ACT-R operations, we have recently turned to EEG and MEG, which provide data at a millisecond-resolution. These experiments have provided evidence for additional processes in the standard fan-experiment: a familiarity process and a more involved decision process. In this talk I will discuss whether these results should lead to changes to the architecture or only to updates of existing models.
Daniel Cassenti (ARL) – Evoked Response Potential Latency Modeling and Production Time Prediction
Although a cognitive process is typically a long sequence of cognitive events (e.g., the sequence of productions in ACT-R), cognitive scientists must infer mental steps based largely on the end points of the process – the stimulus and response. This presentation will examine the relationship between Evoked Response Potentials (ERPs) and the cognitive events they signify, using empirical data to segment between the start and end of a cognitive process. Further, I will examine how to implement these ideas into ACT-R by describing three models. With the successful inclusion of brain localization in ACT-R, this presentation will lay out the case for why it is important to incorporate temporal properties of brain events as well. A program of research is proposed to help improve production time estimation in ACT-R.
Andrea Stocco (Washington) – Implications from a Dynamic Causal Modeling Analysis of Brain Data
In the canonical association between modules and brain regions, ACT-R’s procedural module has been mapped to the basal ganglia. This mapping has found a number of experimental verifications with fMRI, and two neural network models have been put forward that provide a robust biological justification for it. There are two potential problems with this. First, the procedural module makes specific and detailed predictions about the directionality of production rules, which have not been tested against fMRI data. The second problem is that the basal ganglia can only perform a subset of the functions of the procedural module. In this presentation, I will try to address these problems by showing a different way of analyzing fMRI data that provides information about the directionality of variable transfer as well as the demand functions of a module. I will then compare the predictions of ACT-R’s procedural module with the results of this analysis, and use the parts where the model predictions do not match to suggest either modified mappings or perhaps simple modifications to the procedural module.
Kevin Gluck (AFRL) – Pace, Persistence, and Scale
I will describe priorities for the future of the architecture as “Pace, Persistence, and Scale.” I will comment on the extent to which there is any progress on these fronts, describe some enduring challenges, and discuss how these considerations relate to issues of robotic autonomy.
Cleotilde Gonzalez (CMU) – Reflections on Unresolved Problems for Cognitive Architectures
The cognitive mechanisms integrated in ACT-R continue to provide robust demonstrations of computational representations of human behavior. In many ways, this is good news: the essence of human behavior across many environments is alike. However, most of our efforts have focused on demonstrating how the existent mechanisms can account for traditional phenomena rather than on developing new mechanisms to address new classes of phenomena. I will reflect on some open problems in the hope of motivating a discussion of how to represent them computationally.
Dan Bothell (CMU) – Changes and updates for the ACT-R Software
I will describe updates to the current software covering both architectural and functional changes. The most notable architectural change is a new option for the credit assignment of rewards provided in the utility learning mechanism. The change is to only apply rewards to the productions that have “completed” all of their actions instead of to all those which have been selected. The most significant change to the functionality is a redesign of the history tools available in the ACT-R Environment to provide a more uniform interface and a way to save that information for later inspection.
Glenn Gunzelmann (AFRL) – The Fatigue Module: Unusual, but Necessary
The focus of this talk will be on a fatigue module that provides the capacity for fluctuations in performance as a function of sleep loss, circadian rhythms, and time on task. The module is unusual relative to other ACT-R modules for several reasons: it has no buffers and is not directly responsible for the information processing capacity of the architecture, it influences parameters in other modules through direct module-to-module connections that are not moderated by central cognition, and is fundamentally about the limitations of human cognition. These features, though unusual, are consistent with the existing literature on fatigue, and add an essential dimension often lacking in computational theories of human cognition but necessary to achieve the vision of unified theories of cognition.
Ion Juvina (Wright St) – Learning to Trust and Trusting to Learn
In a series of related projects, we study how trust mediates learning and how trust itself is learned during strategic interaction. We describe an ACT-R model of trust dynamics that accounts for learning within and between the games Prisoner’s Dilemma and Chicken, and a series of validation studies aimed at expanding the range of conditions and tasks to which the model can be applied, including team-based learning. Our work on improving the original model poses fundamental questions about the architecture such as how models can represent other models and learn to interact with them. Complex interactions between trust and learning can be best studied in a cognitive architecture such as ACT-R, which already includes a variety of learning mechanisms. However, we found that new equations and mechanisms need to be developed to deal with the intricacies of trust development, calibration, and repair, including a trust update equation that provides a unified account for a number of effects from the trust literature.
Bill Kennedy (GMU) – ACT-R+: Including Social and Emotional Cognitive Functionality
ACT-R does not provide an implemented theory of social or emotional cognition, i.e., beyond rational cognition. Humans are particularly good at social cognition, specifically, identifying animate behavior and reading the minds and simulation of other agents. These capabilities have been localized in the brain, but that functionality is not yet provided within ACT-R. While the architecture includes a very effective model of memory including similarity and priming effects, these capabilities do not include the effect of emotional valence on memory, necessary to explain the experimental findings. This talk will describe these additional capabilities within ACT-R and their application to human phenomena.
Othalia Larue (Wright St) – From Implicit Affect to Explicit Emotion
We propose a new architectural mechanism to be used for modeling affective states in ACT-R. Some of the existing approaches to modeling affect are inspired by appraisal theories and tend to focus on explicit representations that are hardwired rather than learned. Other approaches attempt to touch upon the implicit aspects of affective processes by investigating the physiological correlates of affect. We propose a solution inspired by the core affect theory, which places implicit visceral reactions to sensory stimuli along two continuous dimensions of affect: arousal (intensity) and valence. We translate the two dimensions in ACT-R as activation and valuation, respectively. The proposed approach unifies the emotion and cognition theories by naturally integrating affect into the existing ACTR mechanisms without requiring additional modules or a major reorganization of the architecture.
Nele Russwinkle (Technical University Berlin) – Spatial Module and Mental Rotation
We extended the architecture with a spatial module and modeled learning and the influence of familiar objects in a mental rotation task. The aim is to predict behavior of people in applied task that depend on spatial competence. What kind of technical support would help them with task, when are errors most probable, in what situation is that task too difficult and the user needs further information.
Troy Kelley (ARL) – Episodic Memory Consolidations: Lessons Learned from a Dreaming Robot
As part of the development of the Symbolic and Sub-symbolic Robotics Intelligence System (SS-RICS) we have implemented a memory store to allow a robot to retain knowledge from previous experiences. As part of the development of the event memory store, justification for an off-line, unconscious memory process was tested. Three strategies for the recognition of previous events were compared. We found that the best strategy used a post-processing process for all memories using pruning, abstraction, and cueing. Pruning removed memories, abstraction used categories to reduce metric information and the cueing process provided pointers for the recognition of episodes. Additionally, we found that post-processing memories for retrieval as a parallel process was the most efficient strategy. This presentation will review the lessons learned from this work and discuss the implications for cognitive architectures.
Matthew Kelly (Carleton) – Holographic Declarative Memory: A Scalable Memory Module
We present Holographic Declarative Memory (HDM), a module that replaces the slot-value strings of ACT-R’s declarative memory with holographic vectors. HDM reproduces the functionality of DM using vectors as symbols, which confers advantages when dealing with large databases. We have demonstrated the suitability of HDM as a substitute for DM on variants of the fan effect task. In HDM, association strengths emerge from the geometries of the vector space. HDM is also scalable: HDM is based on previous holographic models that have been used to infer the semantics of concepts from large untagged corpuses. Finally, HDM have been used in neurally plausible cognitive architectures.
Michael K. Martin, Christian Lebiere, MaryAnne Fields & Craig Lennon (CMU) – Learning Category Instances and Feature Utilities in a Feature-Selection Model
We describe a Feature-Selection model that combines the instance-based learning paradigm (to categorize objects defined by configurations of features) with production utility learning (to learn a subset of features relevant to the categorization). The model is intended to eventually serve as a general model that helps anchor perceptual labels in autonomous systems. Although feature selection and categorization are often addressed separately in machine learning projects, they can be integrated in a cognitive architecture such as ACT-R as a combination of mechanisms including blending, flexible chunk types and and reinforcement learning. We will discuss architectural issues including controlling the feedback loop between declarative and procedural memory, adjusting the level of noise with experience, learning across module boundaries, and accuracy-efficiency tradeoffs.
Frank E. Ritter (Penn State) – Modeling Novice to Expert Performance with a Modeling Compiler
In this talk I will present high-level behavior representation languages in general and one in particular (called Herbal) that helps model performance on a spreadsheet task. The task takes novices about 25 minutes to perform and experts about 18. We created a tool to author models from a hierarchical task analysis. The tool creates 12 models, a completely novice model that has to proceduralize everything, and then 11 levels of initial expertise, ranging from basically a novice to someone that can do the task using just procedures. The models predict the novice to expert transition, and were created particularly quickly. The models fit the data surprisingly well, which suggests that we can now model novices and their learning using a GOMS-like model, and that behavioral modeling languages should be used more.
Niels Taatgen (Groningen) – Towards Persistent Cognition
The true potential of cognitive architectures is to generalize over multiple tasks and domains of cognition. However, research in cognitive architectures mainly consists of building models of particular experimental tasks, and therefore has many of the limitations of standard cognitive psychology research that were lamented in Newell’s 20 questions paper. In order to achieve a truly integrated cognitive theory, a number of additional pieces of theory are necessary. The first is knowledge transfer: how can procedural and declarative knowledge be used for more than one task? With PRIMs, I have implemented a set of mechanisms for procedural transfer between tasks. However, this is only a first step towards an architecture that can contain knowledge of many different tasks, and that is capable of learning additional tasks by itself. For this we need mechanisms that are able to specify and prioritize goals, and mechanisms that can learn new tasks from examples, instruction or exploration.
Dan Veksler (ARL) – Simple Task-Actor Protocol: Model Reuse Across Tasks, Task Reuse Across Models
One of the benefits of employing computational process models in Cognitive Science is that such models can perform the same tasks as human participants. Unfortunately, task interfaces do not often lend themselves to be easily parsed by computational models and agents. Even task software written specifically for the purposes of behavioral simulations is often limited in that it is tailored for a specific modeling framework, making it difficult to do cross-framework model comparison. Simple Task-Actor Protocol (STAP) is a basis for task-development methodology that makes psychological experiment software easier to develop, to connect to computational models, and to set up for data logging and playback. STAP-compliant task development enables model-code reuse across tasks, and task reuse across models.
David Peebles (Huddersfield) – Methods for accelerating ACT-R model parameter optimisation
I describe two methods for ACT-R model parameter optimisation that allow the search of a multidimensional parameter space using populations of models. The first, differential evolution, is a simple, general purpose algorithm that employs an iterative process of mutation, recombination and selection on a population of candidate solutions to converge on a global optimum. The second method employs HTCondor, an open source, cross-platform software system designed to enable high throughput computing on networked computers. HTCondor facilitates parameter search by allowing modellers to run large numbers of instances of the same model in parallel. I will describe the basic principles of differential evolution and HTCondor and draw upon my experience with both to demonstrate how they can be used to accelerate the development of ACT-R models.
Lael J. Schooler (Syracuse) – Cognitive Costs of Decision Making Strategies: A Componential Analysis
Several theories of decision making contend that the use of decision strategies can be determined by the mental effort required. But how to measure the effort—or cognitive costs—associated with a strategy? Previous analyses have mainly focused on the number of attributes used and the type of information aggregation. We propose an approach based on the ACT-R cognitive architecture, called the Resource Demand Decomposition Analysis, that quantifies the time costs of strategies for using specific underlying cognitive resources and takes into account interactions between processing operations as well as the possibility of parallel processing. Using this approach, we quantify, decompose, and compare the time costs of two prominent decision strategies, take-the-best (TTB) and tallying (TALLY). Our results show that claims about the “simplicity” of decision strategies need to consider not only the amount of information processed but also the cognitive system in which the strategy is embedded.
Dan Veksler (ARL) – How Persuasive is a Good Fit of Model to Data: Model Flexibility Analysis
A good fit of model predictions to empirical data is often used as an argument for model validity. However, if the model is flexible enough to fit a large proportion of potential empirical outcomes, a good fit becomes less meaningful. Model Flexibility Analysis (MFA) is a method for estimating the proportion of potential empirical outcomes that the model can fit. MFA aids model evaluation by providing a metric for gauging the persuasiveness of a given fit. MFA is more informative than merely discounting the fit by the number of free parameters in the model, as the number of free parameters does not necessarily correlate with the flexibility of the model. We contrast MFA with other flexibility assessment techniques, provide examples of how MFA can help to inform modeling results, and discuss a variety of issues relating to the use, disuse, and misuse of MFA in model validation.
Robert West (Carleton) – Using Macro Architectures to Scale Up to Real World Tasks
Scaling up cognitive models for application to real world sociotechnical systems presents several challenges. The first is that building these models from scratch is prohibitively time consuming. The second is that there are different ways to build models of the same task; the more complex the task, the more different ways there are to model it. The idea of a macro cognitive architecture is based on the claim that people tend to use their micro cognitive architecture (e.g., ACT-R) in consistent ways to solve high-level problems that occur across tasks, including problem solving, navigation, competition, negotiation, and planning. SGOMS is a macro cognitive architecture for modeling how people use expert knowledge in multi-agent, dynamic environments with interruptions and re-planning. It is implemented in ACT-R and provides a template for quickly adding task-specific knowledge to create a complex model. Thus it solves two problems: how to jump-start a complex model and how to deal with specific high-level macro cognitive issues. This talk discusses some of the issues and lessons learned in creating and testing SGOMS and how we might develop a suite of ACT-R-based macro cognitive architectures.